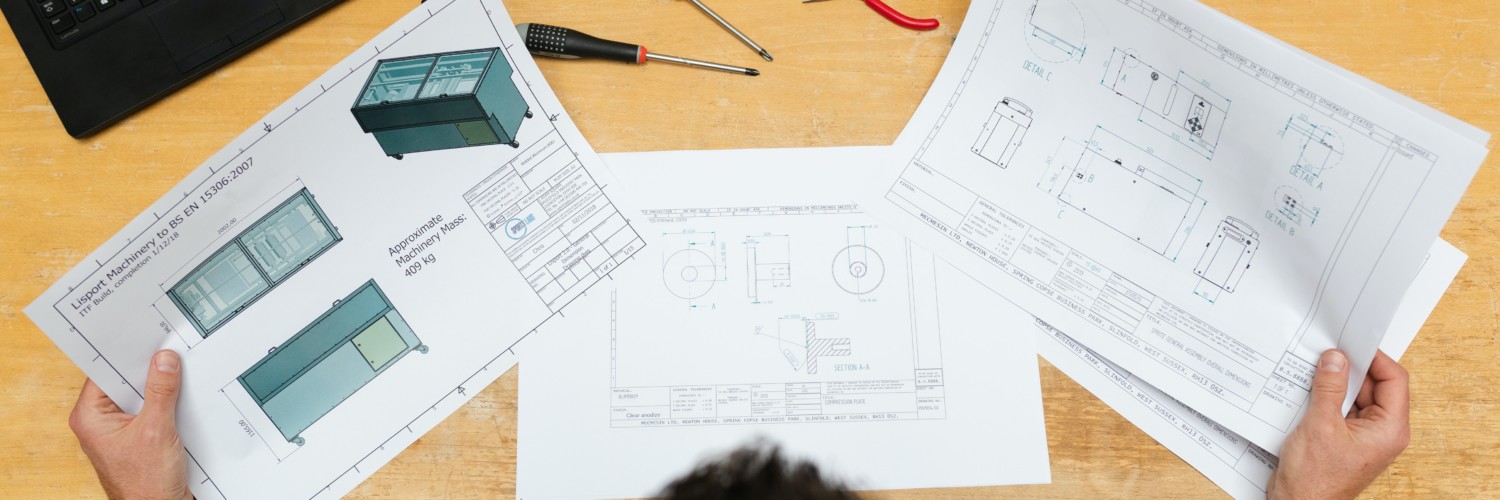
Unlocking the Power of ChatGPT for Rapid Requirements Extraction
Requirements extraction is a critical process in engineering that involves analyzing and interpreting complex technical documents to identify and prioritize the key features, functions, and constraints of a product or system to be designed. However, the traditional methods of requirements extraction can be time-consuming, error-prone, and inefficient, leading to delays, cost overruns, and suboptimal performance.
Recent advancements in natural language processing and artificial intelligence have made it possible to automate and streamline the requirements extraction process, leading to faster and more accurate results. One such technology is ChatGPT, a state-of-the-art language model that can generate human-like responses to natural language queries and tasks, such as text summarization and question-answering. In this blog post, we will explore how ChatGPT can be used for requirements extraction in engineering, and how it can help engineering teams to optimize their product development lifecycle and stay ahead of the competition.
To interact with large language models such as ChatGPT, we use natural language by providing a prompt and requesting the model to generate a completion. This prompt could be in the form of a question, conversation turn, or pattern to extend, among others. However, this interaction is limited to the knowledge the model has learnt during training. Hence, a roadblock for use in requirements extraction. There are at least two ways to handle this roadblock:
- Copy and paste external data into the chat interface for ChatGPT, however there is a limit to how much text can be used in the input. Often the documentation from which requirements are extracted does not fit within this limit.
- Use a framework called LangChain to solve this “limit problem” which can be used to handle large file formats and data sets. Here we split the text into several chunks and still retain meaning, create embeddings and then store these embeddings as an external knowledge base. Next, only the context-related chunks are then retrieved and combined with the question and sent to the large language model (GPT – 4) to generate a response that is contextual and appropriate. The figure below shows how this can work using Azure Cognitive Search and Azure OpenAI Service.
An added advantage is the explainability through citation where the response from ChatGPT also includes citation with a link to the source content for reference.
Additionally, ChatGPT can also assist in identifying potential ambiguities or inconsistencies in requirements by offering alternative phrasings and scenarios. Overall, ChatGPT can be a valuable asset in the requirements engineering process, saving time and increasing the accuracy and completeness of requirements.
ChatGPT in action:
Following is an extract of a contract at:
DDaT22481 - CMMS/EAM Software for the ISIS Facility Description The final date and time for the submission of bids is Tuesday 11th April 2023 at 11:00. DO NOT apply directly to the buyer. All tender information MUST be submitted through the Jaggaer eSourcing Portal. Brief Description of Requirement STFC's ISIS facility uses a particle accelerator to produce neutrons and muons which are used to carry out research in the physical and life sciences. The facility uses cutting edge technology and equipment to operate the accelerator continuously through user cycles, with planned maintenance periods approximately four times per year. The facility operates twenty-four hours per day during the user cycle. To ensure high availability and reliability of the assets within the accelerators, target stations and beamlines we require a software based CMMS / EAM system to store asset information, including its location which may be mobile, maintain the stores inventory and operate a maintenance and work order management system. The scope for the computerised maintenance management software (CMMS) package is as follows: - Plan a broad range of maintenance activities from reactive through to preventative and reliability centred. - Schedule and assign maintenance activities, including shutdown scheduling. - Manage the assets lifespan through their entire lifecycle. - Track assets that are movable in their location. - Management of assets, spares, stores functionality. - Produce reports and dashboards associated with asset activities. - Associate safety procedures and documentation such as RAMS, PTW and LOTO. - Shift Log allowing events during a shift to be recorded and exchanged between shifts and the equipment owners. - Manage the calibration activities and analyse calibration data of assets through their lifecycle. - Connect, communicate, and export data with third party software such as condition-based monitoring systems, spreadsheets, and external reporting software such as Power BI. Please ensure you review all attached information to ensure a full understanding of this requirement. All attachments can be found with the Supplier Attachments tab within the Jaggaer eSourcing Portal. This contract will be awarded based on the evaluation criteria as set out in the RFP document. When this contract was ingested into the GPT-4 model with a question to list functional requirements from the RFP, the resulting list produced were
When this contract was ingested into the GPT-4 model with a question to list functional requirements from the RFP, the resulting list produced were
Furthermore, business development executives can benefit from the increased adaptability of seeking information beyond their current knowledge. For example, they can request further details on RAMS, PTW, and LOTO from GPT-4.
However caution must be taken to verify the same information, if the terminologies are completely unknown to them.
Contact us to find out Solita’s complete offering in Generative AI.
Author: Karthik Sindhya, PhD, AI strategist, Data Science, AI & Analytics,
Tel. +358 40 5020418, karthik.sindhya@solita.fi